Écrit par
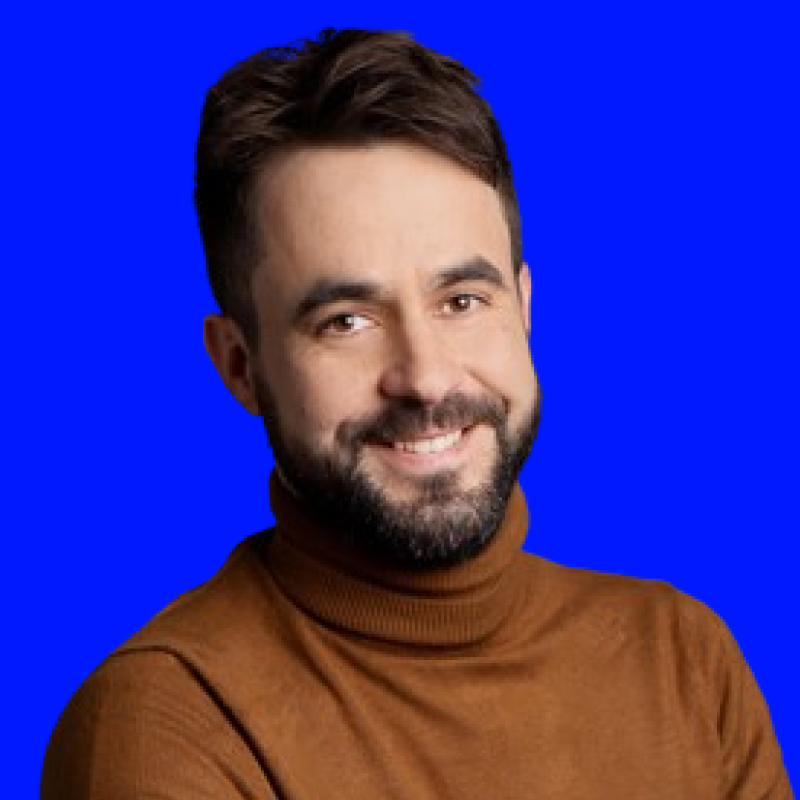
Alexis Laporte
2x Cofounder - 1x Board Member - Tech Specialist
Subscribe to newsletter
Thank you! Your submission has been received!
Oops! Something went wrong while submitting the form.
The principle of a agent IA is to act for the user account, either in its own environment (remote AI agent), or directly on the client's environment (local AI agent).
For example, OpenAI Operator works both as a remote agent from OpenAI servers and as a local agent on macOS, where it can interact with other applications. Many other agents, like Deep Research, are also taking a remote approach.
Remote agents are the most common today because they are easier to design and do not require local installation. However, local agents have a long history in the sector, especially in the form of Robotic Process Automation (RPA).
The Artificial Intelligence sector must take into account several fundamental differences between local and remote agents:
• Confidentiality and control: A local agent provides a greater sense of confidentiality and better risk control by the user.
• Efficient performance: Locally, the agent can run LLM calculations on the user's machine, potentially speeding up machine processing.
• Hardware constraints: A local agent can be limited by the power of the device, unlike remote agents who rely on servers.
This opposition recalls that of the 1970s between mainframes and the emergence of the personal computer (PC), introduced by Hewlett-Packard and IBM (linkage).
The major challenges for AI agents, whether local or remote, are their ability to authenticate themselves for the user. Identifiers are generally stored in managers integrated into operating systems (iOS, Android) or browsers (Chrome). Third-party solutions like 1Password or LastPass are also used.
However, Apple and Google restrict access to these identifiers to protect their ecosystem. This makes it difficult to integrate third-party AI agents like Operator from OpenAI.
This strategic problem is pushing several startups to explore independent alternative processes. For example, Jony Ive is working on a new machine with OpenAI (source).
Take the example of a user asking their AI agent to buy a train ticket:
1. Offer selection: The agent must be able to compare several options and data (example: a €97 train at 9:32am versus. a €32 train at 8:46am).
2. Payment validation: Once the decision is made, the AI agent must access the payment data and potentially interact with the bank.
Card payment can be done in three different processes:
• Manual entry: The user enters his data and validates with his bank.
• Autofill: The OS or the browser automatically fills in the payment fields.
• Third-party system: Providers such as Klarna, PayPal or Apple Pay manage payments without requiring direct identifiers.
An AI agent should be able to automate the entry of payment data or integrate with a third-party system. However, this raises several challenges:
• Security and confidentiality: The storage of identifying data and their access must be protected from the risk of fraud.
• Platform restrictions: Apple and Google may prevent access to ID managers for strategic reasons.
• Bank confirmation: Some payments require strong authentication, making automation more complex.
Thus, although agent payment represents a real positive change, its adoption will depend on the ability of Artificial Intelligence agent publishers to circumvent these technical and regulatory obstacles.
The rise of AI agents in online navigation and transactions raises major strategic and technical questions. It is now crucial to prepare agent navigation paths, especially for SEO and payment. In these paths, the real objective is to identify that an agent, and not a human, is browsing the site and to simplify the task by delivering appropriate content.
• Offline mode: The agent will be able to provide data on the user according to their permissions. This will allow for increased personalization of the offer. It is therefore essential to monitor the evolution of AI agents and to get closer to publishers.
• Connected mode: When paying by card, the agent will fill in the fields automatically. However, it is relevant to rethink the shopping and shopping cart process. For example, allowing the user to complete the purchase later while keeping track of the initial attempt made by the agent.
• If a third party service (like Klarna or PayPal) is used, it is likely that the agent has access to the user's credentials.
• However, it is crucial to provide specific access for agents, involving a quadripartite authentication method: the merchant, the user, the payment service and the agent publisher.
• Working beforehand with the publishers of payment solutions will facilitate this integration.
Agentic payment opens up new perspectives in automation transactions, but remains a technical and strategic challenge. Les undertakings must prepare now by optimizing their shopping experience and by collaborating with the editors of Artificial Intelligence agents to adapt to this transformation of online commerce.
Join our corporate venture building club